Music Can Be Infectious Like a Virus
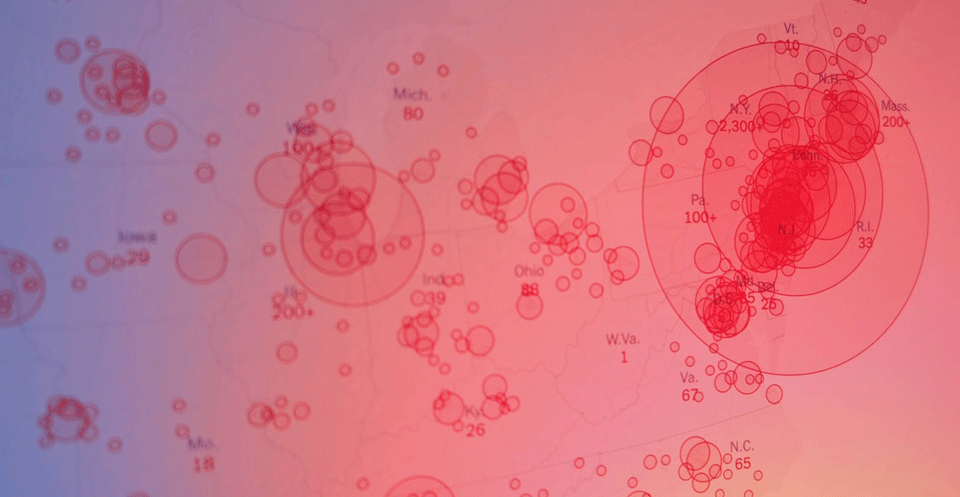
In this article, we learn that a close knit community will not only pass along germs more easily, but music too…
Modelling song popularity as a contagious process
Abstract
Popular songs are often said to be ‘contagious’, ‘infectious’ or ‘viral’. We find that download count time series for many popular songs resemble infectious disease epidemic curves. This paper suggests infectious disease transmission models could help clarify mechanisms that contribute to the ‘spread’ of song preferences and how these mechanisms underlie song popularity. We analysed data from MixRadio, comprising song downloads through Nokia cell phones in Great Britain from 2007 to 2014. We compared the ability of the standard susceptible–infectious–recovered (SIR) epidemic model and a phenomenological (spline) model to fit download time series of popular songs. We fitted these same models to simulated epidemic time series generated by the SIR model. Song downloads are captured better by the SIR model, to the same extent that actual SIR simulations are fitted better by the SIR model than by splines. This suggests that the social processes underlying song popularity are similar to those that drive infectious disease transmission. We draw conclusions about song popularity within specific genres based on estimated SIR parameters. In particular, we argue that faster spread of preferences for Electronica songs may reflect stronger connectivity of the ‘susceptible community’, compared with the larger and broader community that listens to more common genres.
1. Introduction
Music is ubiquitous in society; everyone listens to it and most people prefer certain styles. This ubiquity results in an enormous variety of music and a huge number of songs for listeners to choose from. In spite of this abundance, a remarkably small number of popular songs are almost immediately recognizable to most people at a given time. How does a song become popular and how is it that certain songs become so much more popular than others? What are the underlying social mechanisms that drive these processes?
There are many similarities between the release of a new hit song and the outbreak of an infectious disease. When an infectious disease first enters a population, it is transmitted from person to person via social interactions. Prevalence eventually reaches a peak and then declines as the susceptible pool is exhausted and/or infectious individuals recover. After a new hit song is released, it also ‘spreads’ rapidly through a population, from person to person and through various media, eventually reaching some peak popularity and then diminishing in appeal. At the end of a disease epidemic, a large proportion of the population will have been infected with the disease, whereas at the end of a hit song’s period of extreme popularity, a large proportion of the population will recognize that song.
Could the same social processes that facilitate spread of infectious disease in a population also drive song popularity? Popular songs are often described as ‘viral’ or ‘catchy’ as if they could ‘infect’ people; perhaps this description is more apt than has been previously recognized. In fact, the download time series for many popular songs that we examine in this study are similar in shape to time series for infectious diseases. This resemblance suggests that it is possible that there are social mechanisms underlying song popularity similar to the social mechanisms that drive the spread of an infectious disease, and has acted as our motivation to investigate standard epidemiological models as a tool to study song popularity.
Here, we consider how well a standard epidemiological model and a purely phenomenological spline can fit download time series for popular songs. For comparison, we also fit the same mechanistic and phenomenological models to stochastically simulated epidemic data. If song popularity is driven by a contagious process, then we would expect a mechanistic epidemic model to perform as well relative to a spline when the two models are applied to song download data as it does when they are applied to infectious disease data. If this is the case, then we can attach meaning to the epidemiological parameters estimated for popular songs based on disease transmission model fits and interpret these parameters to draw mechanistic conclusions about song popularity, which we cannot do with purely phenomenological models like splines.
We study data from a large and detailed database of song downloads from 2007 to 2014, a period when downloading (as opposed to streaming) was a primary method of music consumption (for further context on downloading versus streaming, see Aguiar).
2. Background
(a) Song popularity research
Song popularity has been the subject of much research. Some authors have sought to predict the peak and duration of a song’s popularity based solely on its previous popularity rankings. Others have attempted to determine what musical features make a song popular. While there may be specific musical characteristics that predict popularity, social processes also affect how a song gains popularity. Given that complex interactions undoubtedly exist between musical and social factors, disentangling the influence of each on a song’s popularity is a difficult task.
Previous research has found both support for and evidence against the idea that musical features can predict a song’s popularity. Nunes & Ordanini used audio information to show that songs that were number 1 hits on the Billboard Hot 100 Charts in the past 55 years had distinctly different instrumentation than songs that never climbed above the 90th position on these charts. Dhanaraj & Logan found that audio and lyric information about a song could each be used to generate better-than-random predictions about whether a song would be a hit. However, in their study of harmonic and timbral trends in the Billboard Hot 100 Charts over the past 50 years, Mauch et al. found that the frequency of specific timbral characteristics cycled in the Hot 100 Charts as musical styles with different types of instrumentation came in and out of fashion. In addition, Pachet & Roy failed to predict songs’ popularity based on audio characteristics, regardless of whether these characteristics were quantified from an audio signal or from human input.
Several studies have found that information from social media sites, social music sites or peer-to-peer file-sharing networks can predict song popularity, which hints at the underlying social processes driving song popularity. Bischoff et al. built a model that predicted song popularity based on various Last.fm tags relating to user listening habits and previous popularity of the artist in question; Koenigstein et al. demonstrated that search queries from Gnutella could be used to predict a song’s peak position in the Billboard Hot 100 Charts. Schedl et al. used Last.fm play-count data to predict popularity of artists in specific countries. They compared this method with predictions based on (i) user posts from Twitter, (ii) information from shared folders in Gnutella, and (iii) the number of pages returned by search engines that were related to an artist in a specific country. Kim et al. also used Twitter posts to predict song popularity, finding that hashtags related to music listening behaviour of users could help forecast rankings of songs on Billboard charts. Zangerle et al. extended this work to look at data over a longer time period. They found that although Twitter data alone were not sufficient to generate good song popularity predictions, using these data in multivariate predictive models significantly increased the models’ predictive ability.
Support for the idea that social interactions have a high impact on song popularity was presented in a study by Salganik et al.. They played the same set of new music for several distinct groups of participants; song popularity was much less predictable, and between-song differences in popularity more extreme, when others’ opinions of songs were presented with the music.
Lastly, researchers have used neural imaging to examine the influence of a song’s overall popularity on adolescents’ rankings of that song. The functional magnetic resonance imaging (fMRI) data collected in this study suggested that teenagers are more likely to change their evaluation of a song to more closely align with its overall popularity rating as a result of the anxiety created by a difference between their opinion and the opinion of others. Neural activity in specific regions of the brain while listening to songs significantly correlated with sales data for that song over the next 3 years, even though subjective ratings of the songs from participants did not.